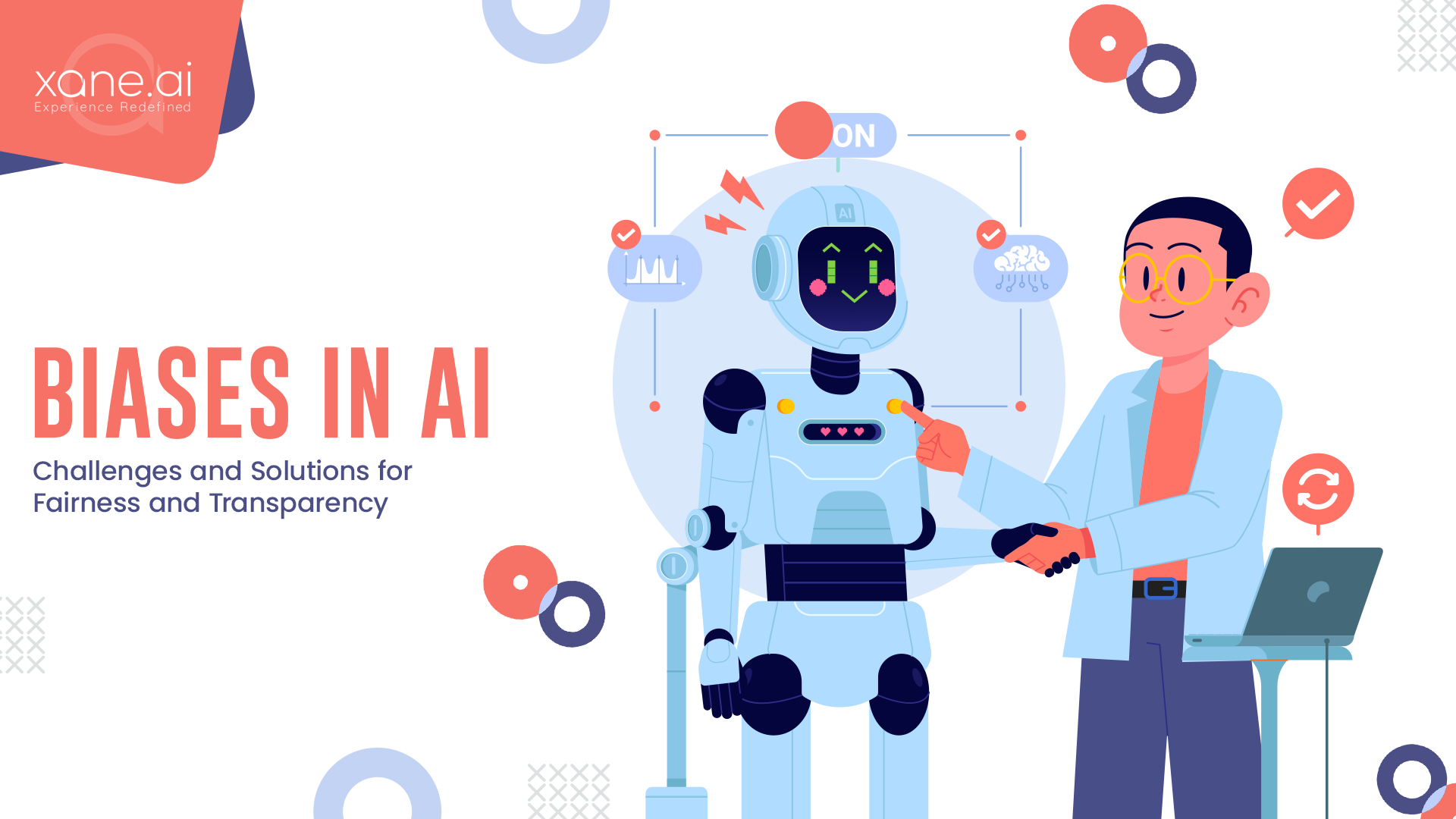
08 Apr Biases in AI: Challenges and Solutions for Fairness and Transparency
Blog by Oluwasegun Oke
As applications of AI-powered machines continue to mount and dominate important domains in our society, noted at a time when profit-driven businesses and individuals are looking to key into its vast potential in implementing and deploying simplified end-to-end personalized solutions that can improve both efficiency and accuracy level of any decision-making process.
Yet inherited fundamental biases from many developers’ ends have stunted the growth of AI systems across different disciplines, as growing concerns over ethical and moral justifications of its use cases – especially behind the unknown process followed in its mechanism during automation have prevented their 360-degree commercial engagement in many fields, including autonomous vehicles, criminal investigation, quality healthcare, and facial recognition systems.
So on the whole, today’s blog is designed to x-ray the emerging challenges of biases from further development of AI systems, or transfer and integration into emerging innovations, as well as the best way forward to discouraging negative integrity or fairness, inequality and lack of transparency in data, so that biases in any case can be fully addressed to forge a positive futuristic path, and prevent any harmful relationship of AI systems with man.
Various Levels of Bias in AI
Even though many AI-powered applications are currently flooding marketplaces, by popular demand, it is understood that a growing number of its solutions is still in the pipeline, or slowing down over transparency issues, due to unwavering concerns from different segments of society, and NGOs, to put security, and safety first, in the adoption of AI systems, to protect the public interest.
What’s more, we will apply the next sections in giving credence to the existence of flawed data and AI biases, while discussing various forms they usually take, and discussing when they pose a threat, plus the techniques and advanced tools that must be incorporated to find a lasting solution.
Different Forms of Bias in AI
Historical Bias
Historical bias includes pre-existing notions, conclusions, practices and norms that have found their way into the processing of sampled data, as they are bound to be integrated into the concerned computer programmer’s work in the form of discriminatory, derogatory, and stereotypical solutions in the outputs of a good number of AI systems.
Nevertheless, the harmful effects of this scourge go to serve only one purpose – extreme and untold hardship – hence distorting the opportunity desks of many underrepresented groups, who are already perceived as inferior by race, age, gender, and societal rankings across many segments of our society.
Representation Bias
A bias picked up during the defining and integrating process of the elements of fairness and transparency while training and simulating specific algorithm models of different contexts and representations.
For instance, it is the same reason Amazon’s facial recognition system once had a hard time detecting dark faces, because most of its data set was trained on features of white faces.
Measurement Bias
When features and labels are being integrated into models, it is important to ensure that the data available are of the highest quality, as well as not being a noisy proxy of the actual data needed. Otherwise, this challenge can be aggravated further, not to add that the process of probing data for bias can be problematic at this stage.
Modelling Representation and Bias
Let’s even assume developers manage to have access to unflawed data for modelling, the modelling methods adopted can as well be a source of bias, and the two ways this threat is widespread are illustrated below:
Evaluation Bias
This is when the benchmark that has been adopted in the process of training models to exhibit the right quality does not properly fit into the functionality of the model or yield to the general population of the developed context and representation.
Aggregation Bias
It takes place whenever heterogeneous populations are unethically combined in the development of predictive models. It helps build up a double standard, in that AI applications once thought to be spot-on and hands-on ultimately come out as lacking depth, being uninspiring, and not even having the previously labelled predictive value.
Introduction of Bias Through Human Reviewers
Even if you manage to get past already highlighted fields an AI system bias can be introduced, and a human reviewer can introduce his own trademark biases to distort previous attainments, by overriding the accurately predicted outputs of the model in question.
What is Fairness?
The definition of Fairness in the AI universe is still in its developmental stages and must be placed, understudied, and implemented from a multi-disciplinary perspective. But let’s take a widely accepted definition:
Fairness is either the absence of prejudice or when there is no preference for an individual or group, based on their given characteristics.
Therefore, in the next sections, we look at different ways computer systems can lose their zero tolerance for bias through various means.
An Example of Fairness in an AI System
Let’s take a very accurate binary classification model as a good example of exhibiting fairness in AI. And we have two distinct groups of yellow and green, representing variables, like genders, race, geographical locations, seasons, festivities, dates, and so on.
The game changer is that aggregation bias may encroach these representations to distort the previously reported predictive value, and raise concerns for bias, and for due fairness to be engaged.
Best Practices to Manage Biases in AI
One way of improving AI systems to develop close to zero bias is to separate each of its model’s groups into their distinct categories, to simplify and distinguish diverse and temporal representations, and in turn define their boundaries, so that their decision-making capabilities are enhanced.
Another best practice is to prevent underestimation or overestimation of any probabilities for different outcomes, by calibrating each group’s predictions, or better still introducing counterfactual fairness techniques to stabilize and consolidate every predictive outcome, no matter the fluctuations or changes taking place across different groups making up the model.